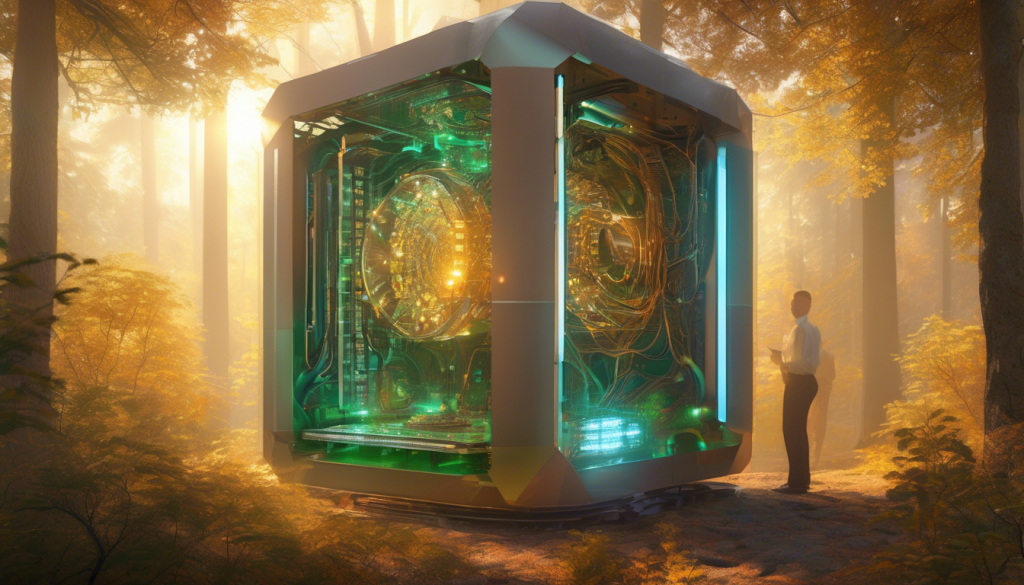
Quantum Machine Learning: Where Computing Power Meets AI Innovation
The fusion of quantum computing with machine learning is creating a technological synergy that promises to solve complex problems beyond the capabilities of classical systems. In 2025, quantum machine learning (QML) stands at a critical juncture where theoretical potential is beginning to translate into practical applications across multiple industries.
The Quantum Advantage in Machine Learning
Quantum computing leverages the principles of quantum mechanics—superposition, entanglement, and quantum interference—to process information in ways fundamentally different from classical computers. When applied to machine learning tasks, these quantum properties can offer significant advantages.
Accelerated Processing and Optimization
One of the most compelling quantum advantages comes in the form of processing speed. IBM and JPMorgan researchers demonstrated that quantum approaches to option pricing could achieve the same accuracy with thousands of samples instead of millions required by classical Monte Carlo methods—a quadratic speedup, as reported by PostQuantum.com.
This acceleration extends to optimization problems central to machine learning. According to a recent survey by SAS, over 60% of business leaders across industries including healthcare, manufacturing, and finance are actively investing in quantum AI precisely because of its potential to provide faster answers to complex questions involving numerous variables.
Enhanced Pattern Recognition
Quantum computing’s ability to exist in multiple states simultaneously (superposition) makes it particularly well-suited for pattern recognition tasks. This capability enables quantum algorithms to explore many potential solutions at once, potentially leading to better workflow optimization and resource utilization than common machine learning algorithms, as noted by TechTarget.
Current Breakthroughs in Quantum Machine Learning
Quantum-Enhanced Generative AI
In 2025, IonQ has made significant strides in enhancing generative AI models through quantum computing. At the Q2B Tokyo conference, IonQ demonstrated new hybrid quantum applications that optimize materials science properties using Quantum-Enhanced Generative Adversarial Networks (QGANs). These applications have shown improved accuracy over purely classical methods in tasks such as sentence sentiment classification, according to IonQ’s announcement.
IonQ’s research revealed that their quantum-enhanced models achieved higher quality scores for synthetic image generation in up to 70% of cases versus classical models, as reported by Green Stock News.
Quantum Computing for Drug Discovery
Perhaps the most promising application of quantum machine learning is in drug discovery. Quantum computing combined with AI is revolutionizing this field by overcoming classical computational limits. As detailed in a recent study, quantum algorithms significantly improve molecular simulations and screening accuracy while reducing computational time.
A real-world example of this breakthrough came from Australian scientists at the University of Sydney, who successfully used a quantum computer to simulate how real molecules behave when exposed to light. According to quantum chemist Ivan Kassal, their approach is “about a million times more resource-efficient, enabling complex chemical dynamics to be studied with far fewer resources than previously thought possible.” This advancement, published in the Journal of the American Chemical Society, has far-reaching implications for designing photoactive drugs, UV-protective materials, and next-generation solar technologies.
Industries Embracing Quantum Machine Learning
Financial Technology
The financial sector is pioneering quantum machine learning adoption. A novel quantum machine learning model called QFDNN (Quantum Feature Deep Neural Network) has been introduced to enhance fraud detection and loan eligibility prediction. This model addresses challenges in processing high-dimensional financial data by offering improved efficiency and noise resilience, achieving competitive accuracies while reducing computational costs, as reported by Quantum Zeitgeist.
Healthcare and Life Sciences
Beyond drug discovery, quantum machine learning is being applied to analyze complex biological data. Wellcome Leap’s Quantum for Bio Challenge has advanced several projects to its final phase, including initiatives focused on drug discovery for conditions like myotonic dystrophy. These projects, involving companies like Quera Computing, aim to demonstrate how quantum computing can accelerate computer-aided drug discovery through quantum simulation, according to Noticias Ultimas.
Manufacturing and Retail
Quantum technologies are enhancing manufacturing and retail operations through more efficient supply chain and inventory management. Quantum computing’s ability to solve complex optimization problems makes it ideal for these sectors, where real-time inventory management and supply chain optimization are crucial.
What This Means For You
For Professionals in AI and Data Science
If you’re working in AI or data science, quantum machine learning represents both an opportunity and a challenge. While it offers powerful new tools for solving complex problems, it also requires new skills and understanding.
Pro Tip: Start by familiarizing yourself with quantum computing concepts through courses offered by institutions like Keio University and The University of Tokyo, which provide quantum computing education through the IBM Quantum Network. According to IBM, these programs offer hands-on quantum programming training using Qiskit.
For Business Leaders
For executives and decision-makers, quantum machine learning could provide competitive advantages in data processing, optimization, and predictive analytics. The SAS survey found that high interest in quantum AI is driven by its potential to provide fast answers to complex questions involving myriad variables.
Getting Started:
- Assess which areas of your business could benefit from quantum-enhanced analytics
- Consider partnerships with quantum computing providers or research institutions
- Start small with hybrid quantum-classical approaches that can show immediate benefits
For Healthcare Professionals
In healthcare, quantum machine learning could accelerate drug discovery, improve diagnostic accuracy, and enhance personalized medicine. The University of Sydney’s breakthrough in simulating molecular behavior demonstrates how quantum computing can model complex, light-induced changes in molecules—essential in processes like photosynthesis, UV damage to DNA, and photodynamic cancer therapies.
Challenges and Limitations
Despite its promise, quantum machine learning faces several significant challenges:
Technical Barriers
Quantum systems are prone to noise, which can significantly affect algorithm accuracy. Most current research on QML algorithms is based on ideal simulations, with only a few studies actually tested on real quantum hardware, according to research from the University of Queensland.
Data encoding scalability remains problematic, requiring hardware capabilities that are not fully developed. This scalability issue is critical for practical applications.
Accessibility Challenges
Quantum computing resources are not as widely available as classical computing resources. This lack of access to quantum hardware and the high cost of using quantum systems make it difficult for most researchers and businesses to fully explore QML.
The need for significant computational resources and specific hardware for quantum simulations can be a barrier. For example, Alice & Bob, a quantum computing company, is investing $50 million to build an advanced quantum lab in Paris with partners Bluefors and Quantum Machines, as reported by Bluefors Oy.
Adoption Hurdles
Most research in QML is focused on theoretical applications, with little practical evidence of its advantage over classical methods in areas like healthcare. This lack of practical applications and real-world success stories can make it difficult to justify the adoption of QML in industries.
The Future of Quantum Machine Learning
Looking ahead, several trends and predictions suggest how quantum machine learning will evolve:
Performance Gains
Quantum AI is projected to achieve a 50-100x performance increase over traditional computing approaches. This could transform areas like drug discovery by simulating complex chemical reactions with unprecedented accuracy, according to Netguru.
Market Growth
The quantum computing market is projected to be between $15–20B by 2025–2030, highlighting the significant growth and investment in this technology. Companies like Classiq, a quantum computing software company, recently raised $110 million in Series C funding to build the software stack for quantum computing, as reported by Constellation Research.
National Initiatives
Countries are investing heavily in quantum technologies. India’s National Quantum Mission (NQM), launched in April 2023, aims to catapult India into the elite league of nations pioneering quantum technologies. With a focus on product development, indigenous innovation, and multi-stakeholder collaboration, the mission has established four technology hubs in quantum computing, quantum communication, quantum sensing, and quantum materials & devices, according to ETGovernment.com.
Preparing for a Quantum Future
As quantum machine learning continues to evolve, professionals across industries should prepare for its impact:
- Develop quantum literacy: Understand basic quantum computing concepts and how they apply to machine learning.
- Stay informed about hybrid approaches: Hybrid quantum-classical systems offer the most immediate practical applications.
- Consider ethical implications: Quantum computing can potentially break current encryption methods, creating security challenges that require quantum-resistant algorithms.
- Explore educational opportunities: Programs like the Vietnam School of Artificial Intelligence and Quantum Computing (VSAIQC-2025) aim to bring students to the frontier of research in quantum computing and AI.
- Monitor industry developments: Companies like IonQ, IBM, and Google are leading quantum machine learning research and commercialization.
Quantum machine learning represents a frontier where computing power meets AI innovation. While challenges remain, the potential benefits—from accelerated drug discovery to enhanced financial modeling—suggest that quantum machine learning will play an increasingly important role in solving complex problems beyond the reach of classical systems.
Are you already exploring quantum machine learning applications in your industry? Share your experiences and questions in the comments below, and let’s continue this important conversation about the future of computing and AI.
Further Reading:
Quantum Computing and AI Integration
The Future of Machine Learning Trends
Quantum Machine Learning: The Interplay Between Quantum Computing and Machine Learning