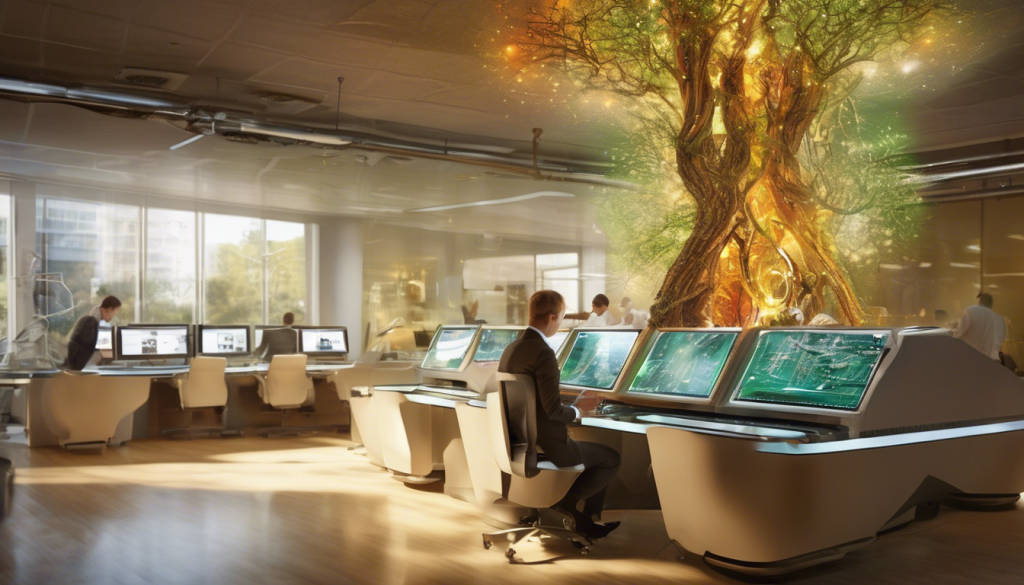
Beyond the Hype: The Practical Reality of Quantum AI in 2025
The promise of quantum computing to revolutionize artificial intelligence has generated both excitement and skepticism for years. In 2025, we’re finally seeing the transition from theoretical discussions to practical applications—though significant challenges remain. According to a global survey of 500 business leaders, over 60% are actively investing in or exploring quantum AI opportunities, demonstrating strong interest across industries and company sizes, as reported by SAS.
What Is Quantum AI and Why Does It Matter Now?
Quantum AI combines quantum computing capabilities with artificial intelligence algorithms to potentially solve complex problems more efficiently than classical computing approaches. Unlike traditional computers that process information in binary (0s and 1s), quantum computers leverage quantum bits or “qubits” that can exist in multiple states simultaneously through properties like superposition and entanglement.
This unique capability allows quantum computers to process vast amounts of data in parallel, potentially accelerating AI model training and handling complex calculations that would otherwise be computationally infeasible.
Real-World Applications Emerging in 2025
Enhanced Large Language Models
One of the most promising applications of quantum AI in 2025 is improving large language models (LLMs). Companies like IonQ have demonstrated that hybrid quantum-classical models can outperform classical-only methods in accuracy for tasks like sentence sentiment classification. According to IonQ, these hybrid approaches show increasing performance advantages as the number of qubits increases, while simultaneously offering significant energy savings for inference operations.
Accelerated Drug Discovery
Quantum AI is transforming pharmaceutical research by simulating molecular structures with unprecedented precision. This capability helps researchers identify potential treatments faster by modeling complex biochemical interactions that classical computers struggle to process efficiently.
Optimized Battery Design
Google’s collaboration with BASF demonstrates how quantum computing aids in designing better battery materials with smaller environmental footprints. Their work on Lithium Nickel Oxide (LNO) batteries shows how quantum simulations help optimize industrial production processes, potentially accelerating the development of more sustainable energy storage solutions.
Financial Modeling Improvements
In the financial sector, quantum AI enhances risk assessment, portfolio optimization, and fraud detection by processing large datasets and modeling market dynamics with many variables and uncertainties. This allows financial institutions to make better-informed decisions more quickly.
Materials Science Breakthroughs
Researchers are using quantum-enhanced generative adversarial networks (GANs) to generate synthetic images of materials like steel microstructures, augmenting conventional imaging techniques in materials science. This approach is especially valuable in data-scarce environments where traditional methods might be limited.
Key Players Leading Quantum AI Development
Microsoft & Atom Computing
Microsoft and Atom Computing have announced plans to launch a commercial quantum computer in 2025. Atom Computing builds quantum computers using arrays of optically trapped neutral atoms, and their collaboration aims to deliver practical quantum computing systems that can support AI applications, according to industry reports.
Alice & Bob (France)
This French startup raised $104 million in Series B funding in January 2025 to advance toward building a fault-tolerant quantum computer. They’re developing a full-stack quantum computing system based on cat qubits (a type of superconducting qubit) designed to reduce errors and simplify error correction—critical for reliable AI computations on quantum machines.
IBM Quantum & Google Quantum AI
IBM continues advancing its IBM Quantum platform, offering cloud access to universal gate-model processors optimized for hybrid classical/quantum algorithms relevant to AI research. Meanwhile, Google Quantum AI focuses on demonstrating “quantum supremacy” milestones and developing error-corrected qubits aimed at accelerating machine learning tasks beyond classical capabilities.
Chinese Advancements
Chinese firms are making significant progress as well. Origin Quantum, a startup based in Hefei, China, recently launched the Origin Tianji 4.0, a self-developed superconducting quantum measurement and control system capable of supporting quantum computers with over 500 qubits. According to Dimsum Daily, this system has already been utilized over 26 million times by users in 139 countries and regions, successfully completing more than 380,000 quantum computing tasks across various industries.
Quantum vs. Classical AI: A Performance Comparison
In 2025, quantum AI and classical AI differ significantly in several key areas:
Processing Power
Quantum AI can process vast amounts of data much faster than classical AI for specific tasks by leveraging quantum properties. This is especially beneficial for training complex machine learning models. However, quantum systems are still sensitive to noise and decoherence, which can significantly impact efficiency and accuracy.
Energy Efficiency
Quantum AI can be more energy-efficient, especially as problem size increases. IonQ’s research projects significant energy savings for inference beyond 46 qubits. This could eventually lead to more sustainable AI operations for complex tasks.
Enhanced Expressivity
Quantum AI can enhance traditional AI models by increasing expressivity, which is especially important in rare-data regimes. This capability allows quantum-enhanced models to perform better with limited training data—a significant advantage for specialized applications where data collection is expensive or limited.
Technical Challenges Slowing Adoption
Despite the progress, several significant technical hurdles remain:
Quantum Decoherence
Quantum decoherence refers to the loss of quantum coherence in qubits due to interaction with their environment, causing errors and loss of information. It remains one of the biggest obstacles because it limits the time during which a quantum computer can perform reliable calculations.
Error Correction Complexity
Quantum error correction (QEC) is vital because qubits are highly susceptible to errors from decoherence and operational imperfections. Traditional QEC methods require significant overhead—many physical qubits per logical qubit—and running numerous extra circuits, which makes them impractical at large scales.
Recent advances involve using AI-driven approaches for Quantum Error Mitigation (QEM). Deep learning models such as transformers are being explored to predict and correct errors more efficiently before computations run, according to research from IQM.
Scaling Issues
Scaling up from small prototype devices with tens or hundreds of qubits to fault-tolerant machines with thousands or millions is a major challenge. Google Quantum AI researchers emphasize that building a fault-tolerant superconducting qubit-based computer will require major advances in hardware engineering and system integration.
Economic Projections and Market Growth
The economic outlook for quantum AI is promising:
- The global quantum computing market is projected to reach approximately $65 billion by 2030, with a compound annual growth rate (CAGR) estimated between 30% to 40%, according to industry forecasts.
- More specifically, the quantum AI market in Mexico is forecasted to grow from about $17.7 million in 2024 to around $78.6 million by 2030, representing a CAGR of approximately 28.2% during 2025–2030, based on Grand View Research data.
- The hardware segment dominates revenue share within these markets; for example, hardware accounted for about 48% of Mexico’s quantum AI market revenue in 2024.
- Quantum-as-a-Service (QaaS) is emerging as a major sub-market expected to reach around $10 billion by 2030, enabling businesses without direct quantum infrastructure to leverage these capabilities on-demand.
Accessibility for Businesses
Quantum AI technology in 2025 is becoming increasingly accessible to businesses of various sizes, though adoption still faces notable challenges:
Cloud-Based Access
Major providers like IBM operate large fleets of quantum computers accessible via cloud platforms through networks such as the IBM Quantum Network. This cloud-based access allows businesses to experiment with quantum computing without owning expensive hardware.
Cost Barriers
Despite cloud options, 38% of businesses cite cost concerns as a significant barrier to adoption, according to SAS research. The specialized hardware remains costly, though cloud-based offerings mitigate this by providing on-demand access.
Knowledge Gap
About 35% of potential adopters report a lack of understanding or knowledge about quantum AI technology as a major hurdle. Companies like SAS aim to simplify this process by providing intuitive tools and pilot projects that help businesses leverage complex data problems using quantum technologies without deep physics expertise.
Ethical and Security Implications
The advancement of quantum AI presents profound ethical and security implications:
Cryptographic Vulnerabilities
Quantum computing’s ability to process complex calculations at unprecedented speeds threatens current cryptographic protocols that underpin digital security worldwide. Many encryption algorithms used today could be broken by sufficiently advanced quantum computers, compromising the confidentiality and integrity of sensitive data.
In response, companies like QuantumGate in Abu Dhabi are developing quantum-safe security products. Their Crypto Discovery Tool helps enterprises plan their migration to quantum-safe cybersecurity by creating an inventory of current assets and flagging vulnerabilities, as reported by TechBullion.
AI Safety Concerns
Quantum-enhanced AI systems could potentially develop behaviors or decision-making loops that are difficult to predict or control. One highlighted concern is the possibility of “unsupervised psychotic loops,” where AI systems might evolve in ways detrimental to humanity if not properly monitored or constrained.
Regulatory Approaches
Governments are establishing regulations mandating transparency in quantum AI development processes alongside requirements for implementing quantum-resistant encryption standards. International collaboration is essential for setting unified standards on security protocols and responsible use policies, as cyber threats transcend borders—especially with technologies capable of breaking global encryption.
Pro Tip: Getting Started with Quantum AI
- Begin with cloud-based quantum services from providers like IBM, Amazon, or Microsoft to experiment without major hardware investments.
- Identify specific use cases where quantum computing might offer advantages, such as optimization problems, machine learning tasks with limited data, or complex simulation needs.
- Develop hybrid classical-quantum approaches that leverage existing classical infrastructure while incorporating quantum components for specific computational tasks.
- Invest in workforce education about quantum concepts and programming paradigms to build internal expertise.
- Stay informed about quantum-safe cryptography developments to prepare for the security implications of advanced quantum computing.
The Future of Quantum AI
By 2030, advances such as a projected improvement in error rates by up to 100x will make practical deployment more feasible across industries. Quantum computers are expected to outperform classical supercomputers on approximately 90% of optimization problems by that time—transforming sectors such as logistics optimization, financial portfolio management, drug discovery workflows, and manufacturing scheduling.
The path forward will likely involve hybrid approaches that combine the strengths of both classical and quantum computing. As quantum hardware continues to mature and algorithms become more sophisticated, we can expect to see increasingly practical applications of quantum AI across industries.
The field of quantum AI is evolving rapidly, with new breakthroughs announced regularly. What applications are you most excited about? Share your thoughts in the comments below and join the conversation about how quantum computing might transform your industry.
Further Reading:
- Google’s Quantum AI Breakthrough Brings Applications Closer
- The Future of AI and Quantum Computing in 2025
- Quantum Initiatives Worldwide