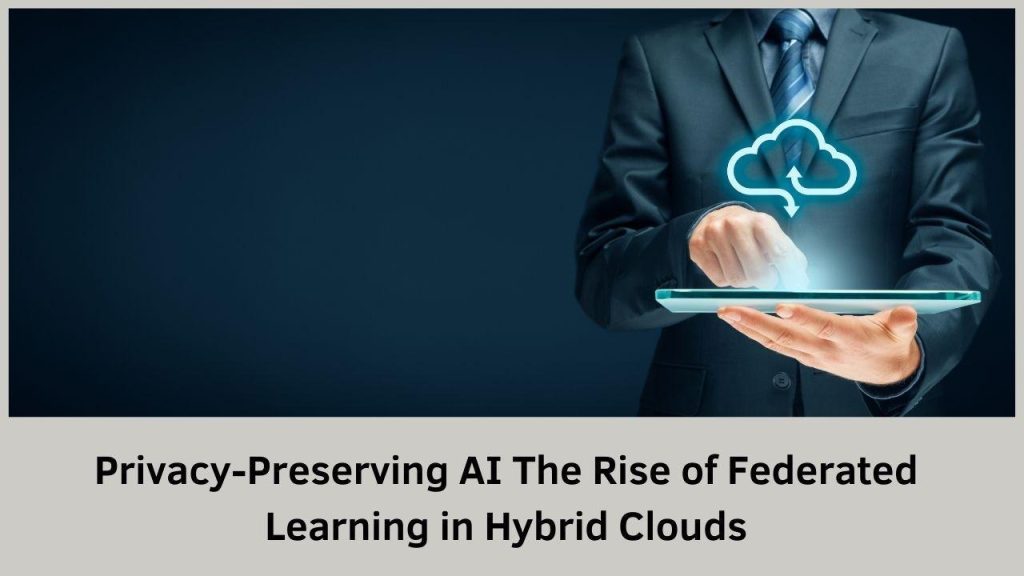
Privacy-Preserving AI: The Rise of Federated Learning in Hybrid Clouds
Author: Angela Scott-Briggs | Chris Lakewoods | Source: TechBullion | Read the full article
In today's world, artificial intelligence (AI) is essential for many businesses, but concerns about data privacy are significant. The article discusses a new approach called federated learning, which allows companies to train AI models without sharing sensitive data. This method keeps data secure and private while still enabling effective machine learning, making it a promising solution for organizations that need to protect their information.
Federated learning works by allowing AI models to learn from data stored in different locations without transferring that data to a central server. This decentralized approach not only enhances security but also helps organizations comply with privacy regulations. The article highlights how this method has led to a notable decrease in privacy-related incidents, making it an attractive option for industries that handle sensitive information, such as healthcare and finance.
The article also emphasizes the benefits of using hybrid cloud environments for federated learning. By combining private and public cloud resources, organizations can improve efficiency and reduce costs while maintaining data privacy. As federated learning continues to gain traction, it is expected to play a crucial role in the future of AI, helping businesses innovate while safeguarding their data.