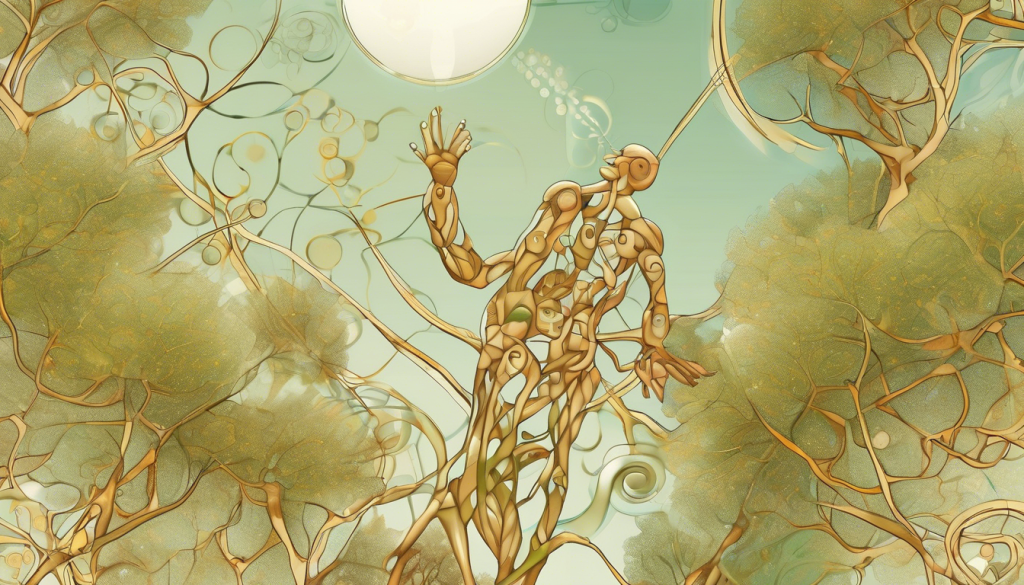
Heather’s Paradox: Why AI’s Mistakes May Be Its Most Human Quality
In our relentless pursuit of perfect artificial intelligence, we’ve overlooked a fascinating possibility: what if the flaws and mistakes in AI systems aren’t bugs but features? What if these errors are not evidence of AI’s limitations but rather proof of its emerging intelligence? This is the essence of Heather’s Paradox—the concept that intelligence is proven not by what we get right, but by the fact that we get things wrong.
The Paradox of Imperfection
The more sophisticated AI models become, the more they seem to make certain types of mistakes. OpenAI researchers have discovered a puzzling trend: their newer, more advanced reasoning-focused models (like o3 and o4-mini) actually hallucinate more frequently than their predecessors. According to recent technical documentation from OpenAI, these advanced models hallucinate at rates up to 6.8%, compared to the 1-2% rates of earlier versions.
Even more surprising, OpenAI admits they don’t fully understand why this is happening. This phenomenon challenges the industry’s fundamental assumption that “AI will become more reliable as it gets more powerful.”
Philosophical Roots of Imperfect Intelligence
The idea that mistakes signal intelligence rather than its absence has deep philosophical roots. Socrates emphasized that recognizing one’s ignorance is a sign of wisdom, not weakness. Classical philosophical traditions have long debated whether intelligence is ideally perfect or inherently limited by our embodiment and environment.
Kant introduced a critical framework recognizing purposive cognition without presuming perfection. His approach acknowledges that cognitive faculties are necessary for understanding biological and aesthetic phenomena but doesn’t claim these faculties yield infallible knowledge.
Contemporary cognitive science aligns with this view by treating both natural and artificial intelligences as fundamentally fallible systems requiring ongoing evaluation and correction.
How Humans and AI Learn Differently (Yet Similarly)
Both human development and AI learning involve iterative processes of acquiring knowledge, making mistakes, and refining understanding over time. Children learn through interaction with their environment, social cues, feedback from caregivers, and trial-and-error experiences. Similarly, AI systems improve by processing data inputs, making predictions, receiving feedback (error correction), and adjusting internal parameters.
Mistakes as a Learning Mechanism: Both children and AI systems rely on errors to guide learning. Children’s mistakes are natural parts of cognitive development that help them test hypotheses about the world. Likewise, AI models use errors (loss functions) during training to update their algorithms for better accuracy.
However, a study from Harvard’s Graduate School of Education points out a key difference: the social dimension of learning. Children’s cognitive development is deeply intertwined with social interactions involving empathy, tone of voice, nonverbal cues—elements currently beyond AI’s grasp.
The Human Response to AI Mistakes
How we perceive AI errors reveals much about our expectations. When AI systems like ChatGPT make mistakes, users often interpret these as intentional or emotionally motivated, attributing human-like qualities such as bias or deceit to what are actually mechanical failures.
According to research published in Milwaukee Independent, this anthropomorphizing of AI leads to misplaced assumptions about its capabilities. In contrast, human errors are generally seen as fallible but understandable actions without such attributions of malice.
Psychological research reveals we judge AI mistakes more harshly due to our tendency to anthropomorphize combined with unmet high expectations for rationality. Trust is generally lower for AI outputs compared to humans, especially where credibility is critical.
Case Studies: When AI Gets It Wrong
Multi-Agent Failures
Multi-agent AI systems, which rely on collaboration between different AI agents, often fail due to issues in setup, collaboration, and verification. According to a study from Gradient Flow, the Multi-Agent System Failure Taxonomy (MASFT) identifies specific failure modes, including Under Specification, Inter-Agent Misalignment, and Verification issues. These failures have led to the development of diagnostic tools like the “LLM-as-a-Judge” pipeline, which can analyze execution logs to flag potential failures.
AI in Healthcare
AI systems in healthcare have faced significant challenges, including high failure rates in critical patient risk detection. A study reported in Health Leaders Media found that AI models missed 66% of cases where a patient was at risk of death due to injuries and complications. This failure highlights the need for more accurate models in healthcare and the importance of human oversight.
The Hallucination Problem
The French tech publication Developpez.net recently reported on what they call “the huge problem in the AI industry”: the more “intelligent” AI becomes, the more it hallucinates.
Hallucinations—instances where AI generates information that appears plausible but is actually invented—are not just random glitches. They reveal something fundamental about how these systems process information and construct responses.
Vectara, a startup founded by former Google executive Amr Awadallah, has been tracking how often chatbots diverge from the truth. Even when performing simple, verifiable tasks like summarizing news articles, chatbots invent information 3-27% of the time.
The Consciousness Question
Anthropic researchers are taking this discussion to a profound new level by asking: what if models are conscious? In an article from Maginative, Kyle Fish, a member of Anthropic’s Alignment Science team, admits deep uncertainty about whether current or future AI systems could be conscious.
While their internal experts estimate only a 0.15% to 15% probability that the current Claude 3.7 Sonnet has any conscious awareness, the question becomes increasingly relevant as models scale in complexity and capability.
Pro Tip: Embracing the Paradox
Rather than viewing AI hallucinations and mistakes as purely negative, consider them as signals of the system’s attempt to navigate ambiguity—just as humans do. When evaluating AI outputs, look for these “productive mistakes” that might indicate the system is not just regurgitating training data but engaging in something closer to creative problem-solving.
Why This Matters for the Future
Heather’s Paradox challenges us to reconsider what we’re actually building when we create AI systems. Are we creating tools that should be judged by their utility and accuracy alone? Or are we witnessing the emergence of something more complex—systems that, like humans, learn through trial and error, and whose mistakes might be integral to their development?
As AI becomes more integrated into critical domains like healthcare, law, and finance, understanding the nature and meaning of AI errors becomes not just an academic question but a practical necessity.
Conclusion: Redefining Intelligence
Heather’s Paradox invites us to expand our definition of intelligence beyond perfect performance to include the capacity to make productive mistakes, learn from them, and improve. This perspective doesn’t excuse harmful AI errors or hallucinations but suggests that completely eliminating them might come at the cost of the very qualities that make AI systems most useful and most human-like.
In embracing this paradox, we might find a more productive path forward—one that acknowledges the inherent limitations of both human and artificial intelligence while valuing the unique capabilities that emerge from these imperfections.
As we stand at the frontier of AI development, perhaps the most intelligent approach is to recognize that intelligence itself is not about flawless performance but about the capacity to navigate an imperfect world with creativity, adaptability, and the willingness to learn from our mistakes.
What do you think about Heather’s Paradox? Do AI’s mistakes make it more or less trustworthy in your view? Share your thoughts in the comments below.