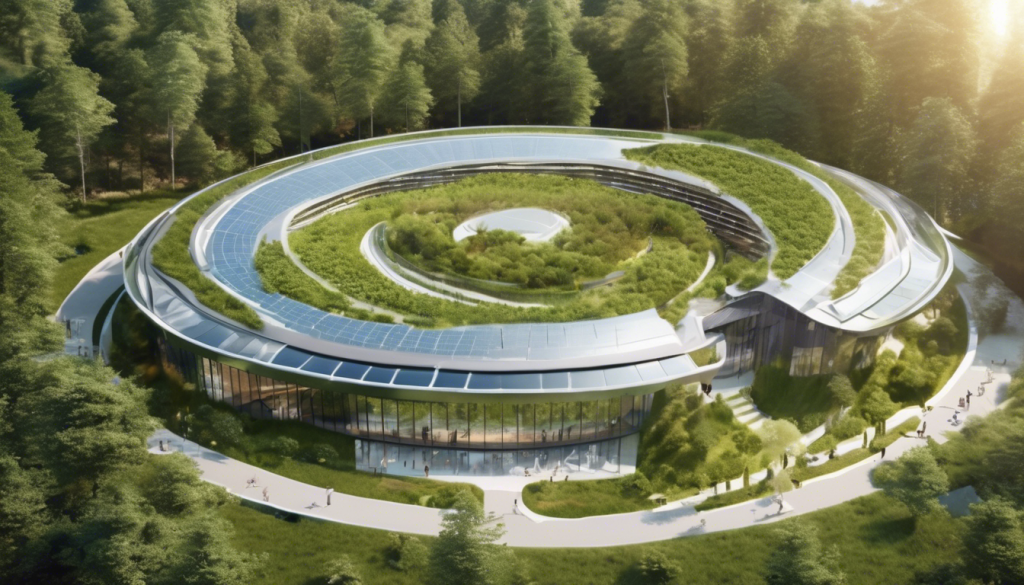
The Hidden Cost of Innovation: Balancing AI Development with Environmental Sustainability
The rapid expansion of artificial intelligence is reshaping our digital landscape, but beneath the surface of technological advancement lies a growing environmental concern. AI systems, particularly large language models, are projected to require approximately 14 gigawatts of additional power capacity by 2030, equivalent to the electricity needs of a small nation. This surge in energy demand raises critical questions about sustainability in an increasingly AI-driven world.
The Carbon Footprint of AI: By the Numbers
AI’s environmental impact extends far beyond what most users see when interacting with applications like ChatGPT or Google’s Gemini. According to the International Energy Agency (IEA), U.S. data center energy consumption is expected to rise from 200 terawatt-hours (TWh) in 2022 to 260 TWh by 2026. To put this in perspective, that’s roughly 1.6 times New York City’s total electricity consumption.
The environmental cost isn’t limited to energy usage. Development Aid reports that training ChatGPT results in nearly 8.4 tons of CO2 emissions—equivalent to the annual carbon footprint of two average American households. This footprint includes not only electricity consumption but also water usage for cooling, rare mineral extraction for hardware, and electronic waste generation.
What This Means For You
Whether you’re a business leader, tech professional, or concerned citizen, AI’s environmental impact has direct consequences for your future. Rising energy demands from AI could lead to increased electricity costs, strained power grids, and potentially slower adoption of clean energy as utilities struggle to meet growing demand.
For businesses implementing AI solutions, understanding these environmental costs is becoming crucial for ESG (Environmental, Social, and Governance) reporting and corporate sustainability goals. As Swiss Re’s Climate Transition Plan demonstrates, major companies are now committing to net-zero emissions by 2050, which will require careful consideration of AI’s carbon footprint.
Industry Leaders Taking Action
Tech giants are increasingly recognizing and addressing the environmental challenges of AI development. Here’s how some leading companies are approaching sustainable AI:
Google’s collaboration with DeepMind has yielded significant improvements in data center efficiency. Their AI-driven cooling system optimization has improved energy efficiency in Google’s data centers by reducing energy waste through neural network models that predict optimal cooling configurations. However, despite these efforts, Computer Weekly reports that Google’s 2023 sustainability report showed a 13% increase in greenhouse gas emissions due to increased data center energy consumption.
Microsoft
Microsoft has committed to becoming carbon-negative by 2030, though its 2023 emissions were 29.1% higher than its 2020 baseline, attributed largely to increased indirect emissions from data center expansion. The company is leveraging AI to enhance operational efficiency across various business functions, with clients like Acentra Health using Azure OpenAI Service to automate tasks, saving significant time and costs, according to Microsoft’s blog.
Alibaba Cloud
Alibaba Cloud has committed to using 100% clean energy by 2030 and has already made significant progress in improving data center efficiency. In the fiscal year ending March 31, 2024, Alibaba reported that their self-built data centers reduced their average power usage effectiveness (PUE) to 1.200, with 56% of electricity coming from clean sources. Their green computing infrastructure enabled clients to reduce emissions by 9.884 million tons in a year.
Strategies for Sustainable AI Development
The path to sustainable AI requires a multi-faceted approach. Here are proven strategies that organizations are implementing:
1. Transition to Renewable Energy
Switching data centers to renewable energy sources is perhaps the most direct way to reduce AI’s carbon footprint. Google plans to operate all its data centers on carbon-free energy by 2030, using wind, solar, and geothermal power, according to The Analyst News.
2. Energy-Efficient AI Models
Developing more efficient AI architectures can significantly reduce energy consumption. Techniques like parameter sharing, model distillation, and hybrid cloud-edge computing can reduce the energy footprint of large language models, as detailed by Indika AI.
Pro Tip:
Consider whether you really need the largest, most powerful AI model for your use case. Smaller, more specialized models often provide comparable results with a fraction of the environmental impact.
3. Carbon Emissions Tracking
Tools like Carbontracker help measure the carbon emissions of AI models during training, allowing developers to monitor and minimize environmental impact in real-time. Companies can also implement internal carbon pricing to measure and offset the environmental impact of their AI systems, promoting energy-efficient solutions.
4. Circular Economy Approaches
AI hardware contributes significantly to e-waste. Embracing circular economy principles—designing for durability, reuse, and recycling of hardware components—can help minimize waste. Companies are exploring closed-loop recycling for rare metals and alternative materials with lower toxicity.
Regulatory Frameworks Shaping Sustainable AI
As AI continues to evolve, regulatory frameworks are emerging to ensure responsible and sustainable development:
European Union AI Act
The EU AI Act, which came into effect in August 2024, is a comprehensive legal framework that categorizes AI applications into five risk levels. While not explicitly focused on sustainability, the Act’s broad approach to ensuring AI systems are safe and responsible can influence sustainable practices by promoting ethical AI development.
United States Regulations
The U.S. is taking a more sector-specific approach to AI regulation. The NIST AI Risk Management Framework, published in January 2023, provides voluntary guidelines for managing AI risks, focusing on reliability, privacy, fairness, and resilience. States like California and Colorado are developing their own regulations, creating a patchwork of laws that businesses must navigate.
United Kingdom Pro-Innovation Approach
The UK emphasizes a flexible, principles-based approach to AI regulation, focusing on safety, transparency, and fairness without comprehensive regulation. This framework allows for sector-specific applications and innovation through “sandbox” environments for testing AI technologies.
Economic Benefits of Sustainable AI
Despite the challenges, sustainable AI offers significant economic benefits and business opportunities:
Market Growth Projections
According to a United Nations report, the AI market is projected to reach $4.8 trillion by 2033, roughly the size of Germany’s economy. AI is expected to drive a $7 trillion to $10 trillion economic expansion over the next decade, potentially adding 10% to global GDP.
Industry-Specific ROI
AI enhances security through fraud detection and predictive analytics in finance, while in manufacturing, predictive maintenance and quality control systems reduce downtime and improve production efficiency. In healthcare, AI improves patient outcomes by enabling earlier disease detection and reducing the cost of late-stage treatments.
Green Technology Market
The green technology and sustainability market, boosted by AI-driven solutions, is projected to reach $73.9 billion by 2030, with a CAGR of 23.7%, according to a Globe Newswire report. Blockchain technology is anticipated to witness the fastest growth in this market due to its ability to enhance transparency and security in environmental initiatives.
Challenges in Implementing Sustainable AI
Despite the clear benefits, organizations face several challenges in implementing sustainable AI practices:
Technical Challenges
- Data Quality and Management: AI systems require high-quality, accurate data to operate effectively. However, data quality issues, such as inconsistent formats and lack of historical data, can hinder AI performance.
- Energy Consumption: Training large AI models requires substantial computational power, leading to high energy consumption and carbon emissions.
- Hardware and Resource Use: The production and disposal of AI-related hardware contribute to electronic waste and resource depletion.
Organizational Challenges
- Initial Investment: Implementing AI systems can be costly, especially for small organizations.
- Workforce Resistance: Resistance from employees can hinder AI adoption. Involving employees early in the implementation process and retraining them for new roles can help mitigate this.
- Sustainability Frameworks: Implementing frameworks like the Sustainable AI Impact Assessment Framework (SAIAF) can help organizations assess and mitigate the unintended consequences of AI on sustainability.
Social and Ethical Implications
The integration of sustainability into AI development involves complex social and ethical considerations:
Equity and Inclusion
Equity is crucial in ensuring that AI benefits are shared across diverse communities, especially those historically marginalized. This involves recognizing and respecting diverse community needs and values in AI development and deployment, as highlighted in a recent University World News article.
Global Impact on Jobs
The UN estimates that AI could impact up to 40% of jobs globally, raising concerns about widespread job displacement. This displacement is not limited to traditional blue-collar roles but is increasingly shifting toward white-collar professions as AI systems grow more sophisticated, according to Tekedia.
International Collaboration
Collaborative efforts among governments, tech companies, and environmental organizations are vital for developing sustainable AI practices that support global sustainability goals. The international community must adopt comprehensive strategies that include energy-efficient technologies and renewable energy sources.
Future Outlook: Emerging Trends in Sustainable AI
Looking ahead, several trends are likely to shape the future of sustainable AI:
AI for Climate Risk Assessment
AI will play a critical role in climate risk analysis and adaptation strategies, helping organizations and governments make informed decisions about sustainability investments.
Sustainable AI Infrastructure
The development of sustainable AI infrastructure, including data centers and manufacturing processes, will become increasingly important to reduce the environmental footprint of AI technologies.
Global Ethical Standards
As AI becomes more integrated into sustainability initiatives, global coordination on ethical standards will be essential to ensure responsible AI development and use.
Getting Started with Sustainable AI
For organizations looking to implement sustainable AI practices, here are some concrete steps to begin:
- Audit Your Current AI Energy Usage: Understand the environmental impact of your existing AI systems by measuring their energy consumption and carbon footprint.
- Set Sustainability Goals: Establish clear, measurable objectives for reducing the environmental impact of your AI operations.
- Choose Efficient Models: Select AI models and architectures that balance performance needs with energy efficiency.
- Partner with Green Data Centers: Work with cloud providers that use renewable energy and have strong sustainability commitments.
- Implement Carbon Tracking: Use tools to monitor and report on the carbon emissions associated with your AI development and deployment.
- Train Your Team: Educate developers and stakeholders about sustainable AI practices and their importance.
Conclusion
The intersection of AI and sustainability presents both challenges and opportunities. While AI’s environmental footprint is significant and growing, the technologies and strategies to mitigate these impacts are evolving rapidly. By adopting sustainable practices in AI development and deployment, organizations can contribute to a more environmentally responsible future while still harnessing the transformative power of artificial intelligence.
The path forward requires collaboration between technology developers, businesses, policymakers, and environmental experts. By prioritizing sustainability alongside innovation, we can ensure that AI’s tremendous potential benefits humanity without compromising the health of our planet.
Have you implemented sustainable AI practices in your organization? What challenges have you faced, and what solutions have you found effective? Share your experiences in the comments below.
Further Reading
- The Comprehensive Guide to Sustainable AI Development
- How AI Contributes to Sustainability in Businesses
- The Impact of AI on the Environment: Challenges and Solutions