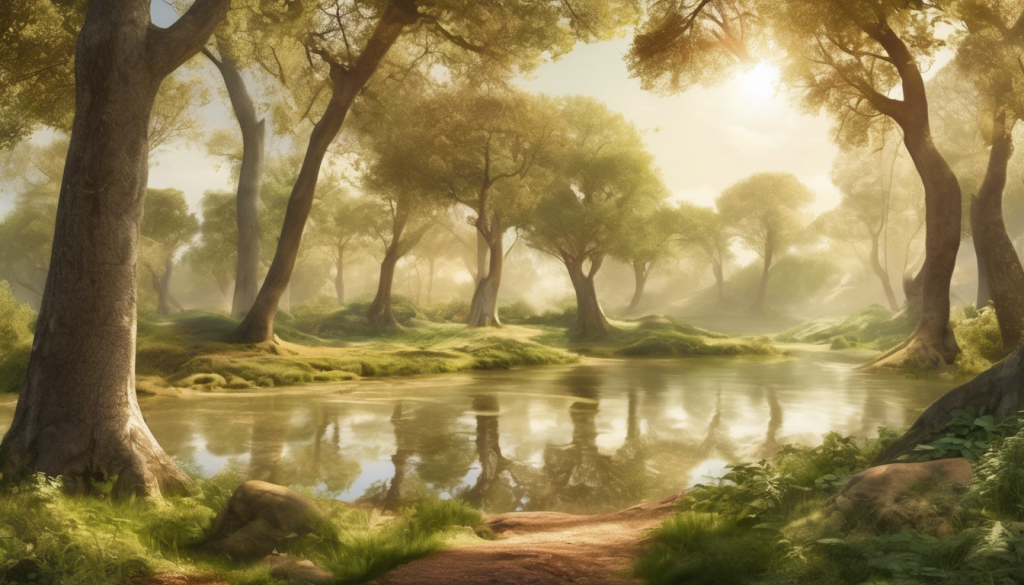
The Evolution of AI: From Historical Milestones to Future Transformations
The Remarkable Journey of Artificial Intelligence
Artificial Intelligence has evolved from a theoretical concept to a transformative force reshaping industries, economies, and daily life. What began as academic research has blossomed into technologies that power everything from the smartphone in your pocket to complex systems managing global supply chains. This evolution represents one of the most significant technological shifts in human history.
According to recent analysis by McKinsey Digital, 72% of organizations now use AI in at least one business function, a dramatic increase from approximately 50% in previous years. This surge in adoption signals we’ve moved beyond the experimental phase into an era where AI is becoming fundamental to business operations.
The Foundation: Early AI Milestones (1940s-1960s)
The journey of AI began with several groundbreaking developments that laid the conceptual and technical groundwork for today’s advanced systems:
- McCulloch-Pitts Neuron (1943): Warren McCulloch and Walter Pitts developed the first computational model for neural networks, establishing the foundation for modern neural network architectures, as documented by DT Labs.
- Turing Test (1950): Alan Turing proposed what would become one of the most influential benchmarks for machine intelligence—a test to evaluate a machine’s ability to exhibit human-like intelligence. This concept, detailed on UpGrad’s blog, continues to influence how we measure AI capabilities.
- Dartmouth Conference (1956): This pivotal gathering officially coined the term “Artificial Intelligence” and established AI as a formal academic discipline, as reported by Grammarly.
- ELIZA Chatbot (1966): Joseph Weizenbaum developed ELIZA, the first chatbot to simulate human conversation using natural language processing, representing an early milestone in human-computer interaction.
Winter and Renaissance: AI’s Path to Deep Learning (1970s-2010s)
AI’s development hasn’t been a straight line of progress. The field has experienced periods of both setback and breakthrough:
- First AI Winter (1970s): After initial enthusiasm, AI faced significant challenges due to overpromised results and limited computational power, leading to reduced interest and funding, as noted in historical accounts on UpGrad.
- Deep Blue (1997): IBM’s Deep Blue defeated chess champion Garry Kasparov, demonstrating AI’s potential in complex cognitive tasks and capturing public imagination about AI’s capabilities.
- Deep Learning Revolution (2006-2012): The field experienced a renaissance with Geoffrey Hinton’s work on deep belief networks, followed by the breakthrough of AlexNet in 2012, which demonstrated the power of convolutional neural networks (CNNs) for image recognition.
Pro Tip:
Understanding AI’s historical cycles of boom and bust can help organizations develop more realistic expectations and implementation timelines for AI projects.
Recent Advances: The Acceleration of AI (2010s-Present)
The past decade has witnessed an unprecedented acceleration in AI capabilities:
- GANs (2014): Generative Adversarial Networks revolutionized AI’s ability to create realistic images and videos, opening new frontiers in creative applications.
- AlphaGo (2016): Google DeepMind’s AlphaGo defeated Go champion Lee Sedol, demonstrating AI’s mastery of a game long considered too intuitive and complex for machines.
- Transformer Architecture (2017): This breakthrough significantly improved natural language processing capabilities, enabling more sophisticated language models.
- Large Language Models (2020-Present): Models like OpenAI’s GPT series have transformed natural language processing, enabling human-like text generation at scale.
These advances have moved AI from specialized applications to general-purpose tools that can be applied across virtually every industry.
Key Players Shaping the AI Landscape
The global AI ecosystem is shaped by a diverse array of companies and organizations:
United States
- Google: A leader in AI development with significant investments in platforms like TensorFlow and specialized AI chips, Google has consistently pushed the boundaries of what’s possible in AI, according to USM Systems.
- H2O.ai: Used by half of the Fortune 500 companies, H2O.ai is transforming businesses with its machine learning platforms.
- Tempus AI: This healthcare-focused company applies AI to combine genomic data with patient records for personalized treatments, as noted by Frank Rimerman.
China
China has emerged as a major AI power with significant government support and innovation:
- Baidu: A leading AI company in China focusing on autonomous vehicles and large language models, Baidu holds a significant market share in China’s LLM market, according to Wikipedia.
- SenseTime: Specializing in intelligent vision technologies, SenseTime has received significant attention for its facial recognition capabilities.
Europe
- Graphcore: Based in Bristol, UK, Graphcore designs AI supercomputers with its Intelligent Processing Unit (IPU), enhancing machine learning model performance.
Regional Variations in AI Implementation
AI adoption varies significantly across regions, influenced by factors such as education levels, economic development, and government support:
United States: Surprising Adoption Patterns
In the U.S., an unexpected trend has emerged where less-educated regions are adopting AI writing tools at a faster rate than more educated areas, according to research from Walk the Street Capital. This challenges traditional assumptions about technology adoption and suggests AI could act as an equalizing force in professional communication.
China: Government-Driven AI Development
China has made AI development a national priority, with significant investments in research and development across various sectors. The Chinese government has created AI innovation zones and funded research, which has propelled China to become a major global AI player. Chinese researchers have developed large language models like WuDao, one of the world’s largest pre-trained language models.
Global Trends
The Asia-Pacific region, including Greater China, has reported some of the largest increases in AI adoption globally. AI implementation is most prominent in professional services, with significant increases in marketing, sales, and product development.
Challenges and Limitations: The Road Ahead
Despite rapid advancement, AI faces several significant challenges:
1. Lack of Context and Common Sense
AI systems struggle with understanding context and common sense, which are essential for making decisions in novel situations. As explained by Adcock Solutions, they are trained on specific datasets and may not apply their knowledge flexibly or creatively, leading to errors when faced with new tasks.
2. Bias and Transparency Issues
AI systems can perpetuate biases present in their training data, leading to unfair outcomes in areas such as hiring, lending, and legal proceedings. The lack of transparency in AI decision-making processes—often described as “black box” problems—complicates understanding and addressing these biases.
3. Data Quality Concerns
The accuracy of AI outputs depends heavily on the quality of input data. Poor data quality, including errors, duplicates, or incomplete information, can lead to flawed insights and decisions, which is particularly concerning in critical sectors like healthcare.
4. Security Vulnerabilities
AI systems are susceptible to manipulation through techniques like adversarial examples, which can cause them to make incorrect decisions. This lack of robustness poses significant security risks, especially in critical applications such as autonomous vehicles or medical diagnosis.
Future Trends: What’s Next for AI?
Looking ahead, several trends are poised to shape the future of AI:
1. Generative AI
Generative AI is revolutionizing content creation across various sectors. According to Synthesia, this technology is being used in applications like AI-powered business consultants, which can analyze business data and suggest strategies for growth. In healthcare, generative AI is accelerating drug discovery by simulating molecular structures and predicting drug interactions.
2. Multi-Modal AI
Multi-modal AI models capable of processing different types of data—text, images, audio, and video—are enabling more comprehensive and human-like interactions. For instance, Google’s Gato is a multi-modal AI system that can perform language, visual, and robotic tasks, as reported by Appinventiv.
3. AI in Healthcare
AI is transforming healthcare through personalized medicine, disease detection, and drug discovery. AI can analyze patient data to recommend tailored treatments and predict disease progression, while in pharmaceutical development, it can accelerate drug discovery by mining large datasets and predicting molecular interactions.
4. Quantum Computing and AI
Quantum computing is poised to revolutionize industries by solving complex problems that are intractable for traditional computers. According to Simplilearn, quantum computing is being applied in areas like cryptography and drug discovery, where it can exponentially speed up processes.
Recent developments illustrate this trend. IonQ, a leader in quantum computing, announced in March 2025 that it has expanded its quantum networking patent portfolio to nearly 400 granted and pending patents. According to a McKinsey report, the total quantum communication market segment size is projected to reach upwards of $14.9 billion by 2035.
5. AI-Enhanced Cybersecurity
As cyber threats become more sophisticated, AI-driven cybersecurity is emerging as a crucial trend. According to Dr. Sherif Abuadbba, Team Leader of Distributed Systems Security at CSIRO’s Data61, “AI is enhancing threat detection, automating defence systems, and enabling predictive analytics, giving security teams the ability to respond faster and more accurately to emerging risks,” as reported by Cyber Daily.
The Growing Importance of AI Education
As AI becomes increasingly integrated into various industries, the demand for AI skills is rising dramatically. According to a recent report highlighted by TechBullion, as many as 2 in every 3 leaders wouldn’t hire someone without AI skills. However, despite over 80% of IT professionals believing they can effectively use AI, only about 12% actually have the skills to create effective prompts for AI systems.
This has led to a 50% hiring gap in AI jobs, emphasizing the importance of proper AI education and training. The report suggests that approximately 70% of global workers need to upgrade their AI skills, particularly in areas like prompt engineering.
What This Means For You
The evolution of AI from theoretical concept to practical technology has profound implications for individuals and organizations alike:
1. Career Development: Acquiring AI skills has become increasingly important across virtually all industries. Consider investing in courses on AI fundamentals, prompt engineering, or AI applications in your specific field.
2. Business Strategy: Organizations should develop clear AI implementation strategies that address both opportunities and challenges, including ethical considerations and data quality issues.
3. Everyday Life: As AI becomes more integrated into daily tools and services, developing a basic understanding of how these systems work can help you use them more effectively and safely.
4. Ethical Awareness: Stay informed about AI ethics and governance developments to understand how these technologies might affect privacy, security, and fairness in society.
The AI revolution is still in its early stages, with many of the most significant developments likely still ahead. By understanding its evolution and staying informed about emerging trends, you can better navigate this transformative technology landscape.
Have you experienced AI’s impact in your work or daily life? Share your experiences in the comments below and join the conversation about how AI is reshaping our world.
Further Reading:
1. The State of AI in 2025
2. AI Trends Reshaping Industries
3. The History and Evolution of Artificial Intelligence