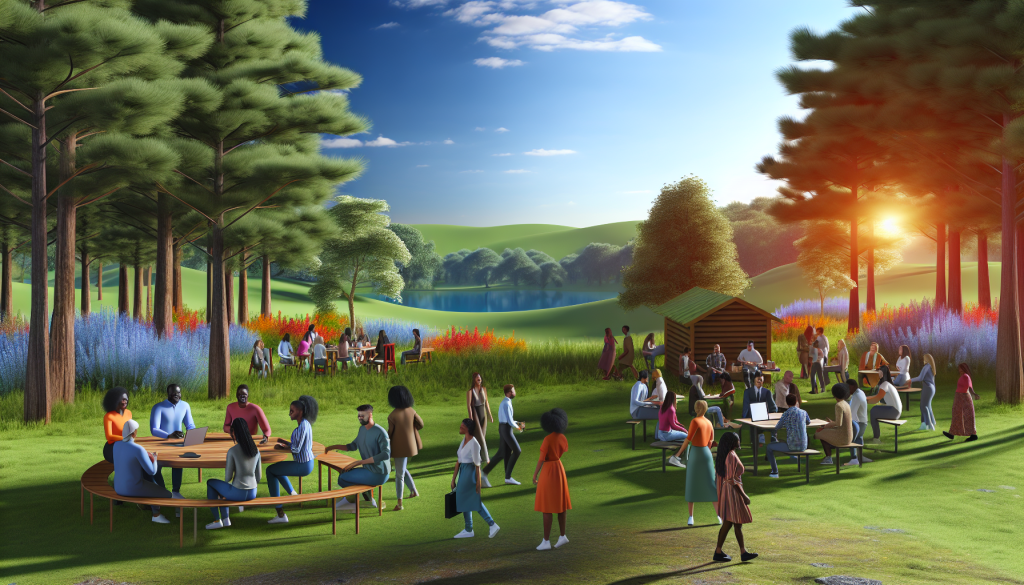
Federated Learning: How AI is Revolutionizing Cloud Security Without Compromising Your Data
The Privacy Paradox: Using AI Without Exposing Your Data
In an era where data breaches make headlines weekly, businesses face a troubling paradox: how to leverage AI's power while keeping sensitive information private. The answer may lie in a revolutionary approach that's growing 456% by 2027.
Federated Learning (FL) is transforming how AI operates in cloud environments, allowing organizations to train sophisticated models without ever moving sensitive data from its source. This breakthrough is particularly crucial for the 78% of businesses that cite data privacy as their primary concern when adopting AI solutions.
"Federated learning marks a transformative shift in AI development, striking a balance between innovation and data privacy," explains Sunil Kumar Gosai, an expert in AI-driven computing whose research is reshaping how we think about secure cloud computing.
What This Means For You
If you're worried about your company's confidential information being exposed through AI training—or facing compliance headaches with regulations like GDPR—federated learning offers a practical solution that doesn't sacrifice performance. Studies show FL implementations have resulted in a 43% decrease in privacy-related incidents, making it especially valuable for those in regulated industries.
How Federated Learning Works (In Plain English)
Traditional AI requires centralizing all your data in one place—imagine sending copies of every confidential document to a third party. Federated learning flips this model:
- Your data stays put: AI models come to your data, not the other way around
- Local training: Models learn from your information where it already lives
- Only insights travel: Just the learnings (not the raw data) move between systems
- Collective intelligence: Insights from multiple sources combine to create smarter models
Pro Tip: Federated learning works particularly well in hybrid cloud setups, where you're already balancing private and public infrastructure. Research shows FL reduces cross-environment data transfers by 78.5%, significantly boosting efficiency while maintaining privacy.
Real-World Success: When Privacy Meets Performance
Healthcare organizations using federated learning have developed diagnostic AI that's 28% more accurate than traditional methods while maintaining HIPAA compliance. Financial institutions can detect fraud patterns across multiple banks without exposing customer transaction data.
In Other Words: You get the collaborative intelligence of pooled data without the privacy risks of actually pooling the data.
The Hidden Cost Savings
Beyond privacy benefits, federated learning delivers surprising efficiency improvements:
- Communication costs cut by 85% through advanced compression techniques
- Central computational demands reduced by 87.3%, lowering cloud infrastructure costs
- Support for up to 15,000 concurrent nodes while maintaining 92% training efficiency
For small business owners and IT leaders operating with limited budgets, these efficiency gains translate to direct cost savings. You can implement sophisticated AI without the massive infrastructure investments traditionally required.
Getting Started with Federated Learning: Your 3-Step Plan
If you're interested in exploring federated learning for your organization, here's how to begin:
-
Assess your privacy requirements: Identify which datasets contain sensitive information that shouldn't leave your environment (customer data, financial records, proprietary information)
-
Evaluate hybrid cloud options: Look for providers that support federated learning frameworks—AWS, Google Cloud, and Azure all offer compatible services starting at $500-1,500/month depending on scale
-
Start with a pilot project: Begin with a non-critical application to test the approach. Most organizations see results within 2-3 months, with minimal disruption to existing workflows
Learning curve: For technical teams already familiar with machine learning, adapting to federated learning typically requires 2-4 weeks of training. Free resources include Google's TensorFlow Federated and OpenFL from Intel.
Looking Ahead: The Future of Private AI in the Cloud
As AI regulations continue to tighten globally, federated learning is positioned to become the standard approach for privacy-conscious organizations. The market for FL solutions is projected to grow at an annual rate of 38.2% through 2027.
Upcoming innovations include:
- Post-quantum cryptography to further enhance security
- Edge-device optimizations for even more efficient distributed computing
- Specialized FL solutions for industry-specific applications
The Balance: Benefits vs. Limitations
While federated learning offers compelling advantages, it's important to understand its limitations:
Benefits:
- Keeps sensitive data secure and private
- Reduces compliance risks
- Improves model accuracy through diverse data sources
- Minimizes bandwidth usage and costs
- Scales efficiently across distributed environments
Challenges:
- Requires more complex implementation than traditional AI
- May experience slower initial training cycles
- Needs careful management of non-uniform data distributions
- Demands specialized expertise to implement effectively
Making the Decision: Is Federated Learning Right for You?
Federated learning makes the most sense for organizations that:
- Handle sensitive or regulated data
- Operate across multiple locations or environments
- Need to collaborate with partners without exposing proprietary information
- Face strict compliance requirements
- Want to reduce data transfer costs
For career professionals looking to stay ahead of AI trends, developing expertise in federated learning represents a valuable specialization. With projected growth rates exceeding 450% over the next two years, skilled practitioners will be in high demand.
Conclusion: Privacy-Preserving AI Is No Longer a Contradiction
The rise of federated learning in hybrid cloud environments demonstrates that privacy and powerful AI aren't mutually exclusive. By keeping data where it belongs while still extracting valuable insights, organizations can navigate the increasingly complex landscape of data regulations while remaining competitive.
Whether you're concerned about compliance, security, or simply want more efficient AI implementations, federated learning offers a practical path forward that addresses the fundamental challenges of modern cloud computing.
As Sunil Kumar Gosai's research highlights, this approach empowers "collaborative intelligence while ensuring robust security," making it a vital technology for forward-thinking organizations in 2025 and beyond.
Related Topics:
- AI-Powered Cloud Security Defining the Future of Cyber Defense
- Lambda raises $480M in funding to expand AI Cloud platform
- Confluent and Databricks Partner to Usher in New Age of Real-Time AI