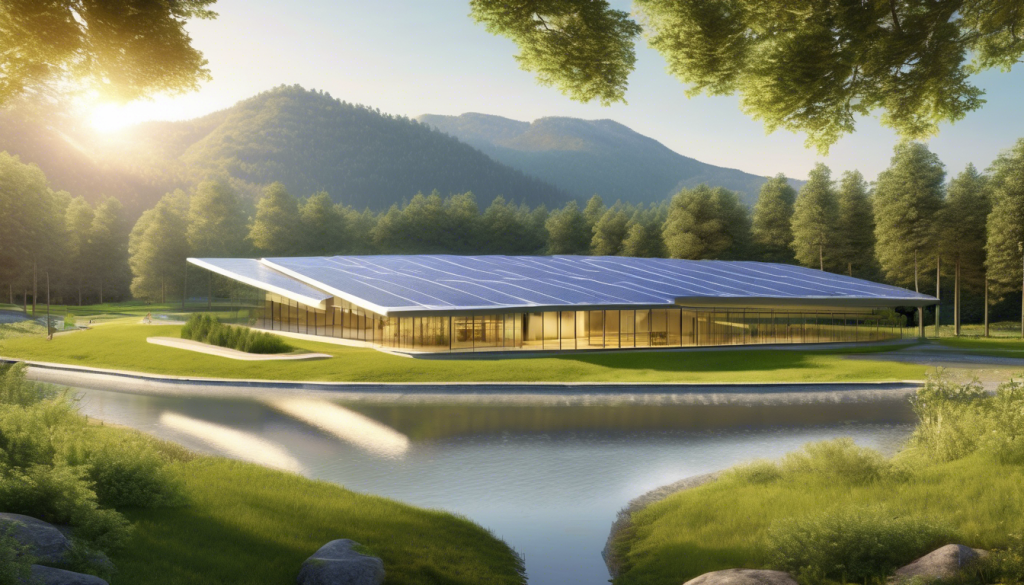
The Hidden Cost of Intelligence: Understanding and Managing AI’s Growing Energy Appetite
The digital transformation powering our economy has a growing appetite that few people see. Behind every AI-generated image, every chatbot response, and every predictive algorithm lies an increasingly significant energy demand that’s reshaping our power infrastructure and raising important questions about sustainability.
Data centers hosting AI workloads consumed approximately 415 terawatt-hours (TWh) of electricity globally in 2024 — equivalent to 1.5% of the world’s electricity use, according to the International Energy Agency (IEA). That might seem modest until you consider the trajectory: this demand has been growing at 12% annually since 2017 — more than four times faster than overall global electricity consumption.
The Scale of AI’s Energy Hunger
The energy intensity of AI operations varies dramatically by application. According to the IEA, a typical language generation query consumes about 2 watt-hours of electricity, while generating a short video requires approximately 50 watt-hours — 25 times more energy. But the real power demands come from the infrastructure supporting these services.
Data centers housing AI systems are undergoing a fundamental transformation in their power requirements:
- Next-generation GPU clusters now demand up to 250 kilowatts per rack, compared to less than 10 kilowatts per rack in 2023
- The largest AI-focused facilities under construction will consume power equivalent to 2 million households
- A typical AI data center requires energy equivalent to powering 100,000 homes
This surge in demand is driving unprecedented infrastructure investments, with tech giants planning to spend $1 trillion on AI data center upgrades, according to Brightlio.
Geographic Concentration Creates Grid Challenges
The distribution of this energy consumption presents additional challenges. Nearly half of U.S. data center capacity is clustered in just five regions, creating localized grid pressures that can strain existing infrastructure.
BloombergNEF forecasts that U.S. data center power demand will more than double by 2035, rising from almost 35 gigawatts in 2024 to 78 gigawatts. This concentrated growth has already led some coal-fired plants to delay closure plans due to rising grid loads from data center expansion.
Environmental Impact Beyond Electricity
The environmental footprint of AI extends beyond electricity consumption:
- Water Usage: Advanced cooling systems in AI data centers require substantial water—about 7,100 liters per megawatt-hour in U.S. centers—exacerbating environmental stress in water-scarce regions
- Electronic Waste: The rapid obsolescence of AI hardware generates significant e-waste, while manufacturing GPUs demands rare earth minerals that deplete natural resources
- Carbon Emissions: Training a single large AI model can produce hundreds of tons of CO2 emissions—equivalent to the annual emissions from driving 123 gasoline-powered cars
According to researchers at Penn State University, GPT-3 training emitted approximately 552 metric tons of CO2, roughly equivalent to 300 round-trip flights between New York and San Francisco.
Projected Growth: A Concerning Trajectory
The IEA projects that global data center electricity consumption will more than double by 2030, from 415 TWh in 2024 to around 945 TWh—comparable to Japan’s entire current electricity usage. This growth is primarily driven by AI’s computational demands.
The most concerning aspect is that this growth may outpace efficiency improvements. Despite hardware efficiency improving by an estimated 350,000x since 2006, absolute demand continues to rise dramatically as models grow larger and applications proliferate.
Strategies for Managing AI Energy Consumption
Major tech companies are implementing various approaches to address rising energy demands:
1. Algorithmic Efficiency and Model Optimization
Companies are developing more efficient AI models that require fewer computational resources. For example, DeepseekV3’s “Mixture of Experts” architecture uses a network of smaller, specialized models working together, promising greater training efficiency according to a Spanish energy publication.
2. Workload Shifting and Geographic Optimization
Cloud providers like Google shift AI workloads to data centers located where renewable energy is most abundant. This strategy helps align high-energy-demand tasks with cleaner electricity sources.
3. Advanced Cooling Technologies
Liquid cooling systems significantly reduce the electricity needed for temperature regulation of servers running AI workloads. This is crucial since cooling can represent a large fraction of total data center energy use.
4. Edge Computing (Edge AI)
Processing data closer to its source (e.g., on local devices) can save between 65% to 80% of the energy compared to traditional cloud-based processing, according to Built In.
5. Integration with Renewable Energy Sources
Tech firms are increasingly powering their operations with renewable electricity, though rapid expansion often outpaces clean energy deployment. Packet Power notes that companies are exploring diverse clean options including solar, wind, and in some cases nuclear or geothermal.
Regulatory Frameworks Emerging
Governments are beginning to address AI’s energy consumption through various regulatory frameworks:
European Union
The EU AI Act, which entered into force in August 2024, imposes requirements on AI systems regarding energy consumption and transparency. It includes provisions for logging AI systems’ energy consumption to increase accountability, according to White & Case.
Additionally, Germany’s Energy Efficiency Act (EnEfG) requires data centers with capacity ≥300 kW to use renewable electricity starting at 50%, rising to 100% by January 1, 2027.
United States
The U.S. currently lacks a national policy framework specifically regulating AI power consumption. Executive orders under the Biden administration emphasize responsible AI development but do not impose direct energy-specific mandates.
Economic Implications for Businesses
The economic impact of AI energy consumption presents both challenges and opportunities:
- Rising Operational Costs: Training large generative AI models can consume enormous amounts of energy—a single model may require around 1,300 megawatt-hours, equivalent to the annual electricity use of about 130 U.S. homes
- Efficiency Gains: While AI increases electricity demand, it also offers opportunities for operational efficiencies that can offset these expenses, particularly in manufacturing, energy, and transportation sectors
- Infrastructure Investment: The global investment in data centers has nearly doubled since 2022, reaching approximately half a trillion dollars in 2024
Emerging Technologies for Efficiency
Several promising technologies are being developed to improve AI energy efficiency:
- Advanced Semiconductor Materials: GaN, SiC, and Graphene offer improved efficiency and thermal performance
- AI-Optimized Cooling: Intelligent cooling systems use real-time data to adjust settings dynamically
- Neuromorphic Computing: Computing based on the human brain’s efficiency could offer more energy-efficient processing models
AI as Part of the Solution
Interestingly, AI itself is becoming part of the solution to energy challenges:
- Grid Optimization: AI models are revolutionizing power grid operations by providing fast, high-fidelity scenario modeling and stochastic optimization
- Renewable Integration: AI analyzes data from renewable sources like wind turbines (which generate over 400 billion data points annually) to optimize performance
- Building Efficiency: Carnegie Mellon University researchers developed an AI agent that continuously monitors sensor data from HVAC systems to detect faults that waste energy
As NREL notes, these applications can help balance the grid’s increasing complexity while supporting the integration of renewable energy sources.
What This Means For You
For businesses and professionals, the growing energy demands of AI have several practical implications:
- Energy costs will impact AI implementation decisions: Consider the total cost of ownership, including energy expenses, when evaluating AI solutions
- Location matters: Geographic areas with abundant renewable energy and strong grid infrastructure may offer advantages for AI-intensive operations
- Efficiency will be a competitive advantage: Organizations that optimize their AI energy usage will have lower operational costs and improved sustainability metrics
Getting Started: Practical Steps
If you’re concerned about your organization’s AI energy footprint, consider these steps:
- Conduct an energy audit of your current AI operations to establish a baseline
- Implement power monitoring solutions like those offered by Packet Power to gain visibility into real-time energy consumption
- Explore edge computing frameworks like TensorFlow Lite for deploying optimized ML models closer to data sources
- Consider workload scheduling tools that align compute-intensive tasks with periods of lower grid carbon intensity
- Invest in efficient hardware and cooling systems designed specifically for AI workloads
The Path Forward
The relationship between AI and energy will continue to evolve rapidly. While current trends show concerning growth in consumption, the technology industry is responding with innovations in efficiency, renewable integration, and system design.
The most sustainable approach will likely combine technological improvements with thoughtful policy frameworks that encourage efficiency without stifling innovation. As businesses and consumers, our choices about which AI technologies to adopt and how to implement them will collectively shape the energy future of artificial intelligence.
As AI continues to transform our world, understanding and managing its energy implications will be crucial for sustainable growth. What steps is your organization taking to balance AI innovation with energy efficiency? Share your thoughts and experiences in the comments below.
Further Reading:
- IEA Report: Energy and AI
- The Impact of AI on Data Center Energy Consumption
- Why AI Uses So Much Energy and What We Can Do About It