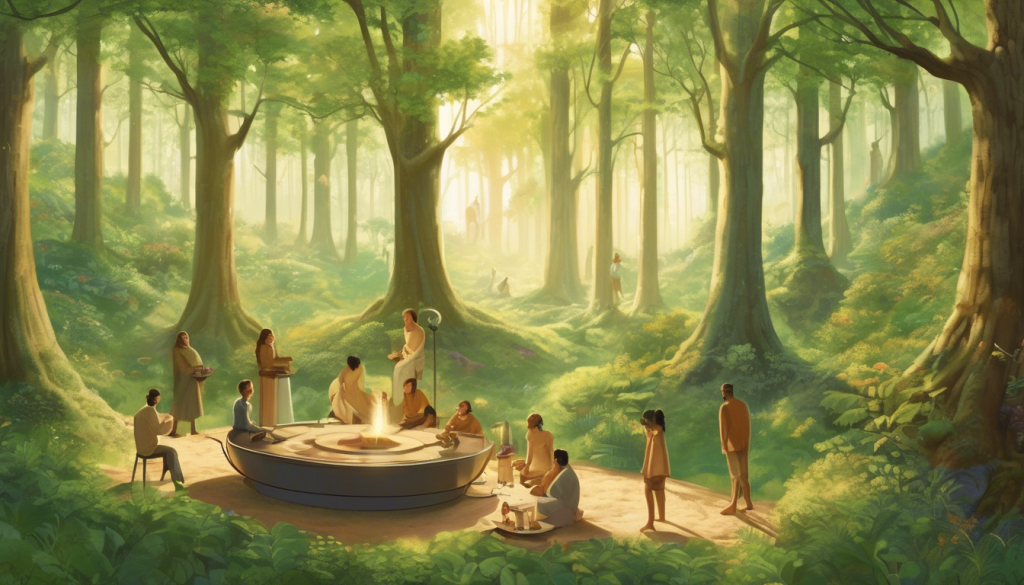
The Human Element in AI Decision-Making: Balancing Automation and Oversight in Critical Industries
The rapid integration of artificial intelligence into critical decision-making processes is transforming industries from healthcare to finance, but with great power comes great responsibility. As AI systems increasingly influence decisions that impact human lives, the balance between automation efficiency and human oversight has never been more crucial.
The Current State of AI Decision-Making
AI adoption across industries has accelerated dramatically in recent years. According to a McKinsey survey, 78% of organizations now use AI in at least one business function, up from 55% just a year earlier. This surge is particularly evident in critical sectors where decisions can have profound consequences.
The financial sector leads this charge, with the banking, financial services, and insurance (BFSI) sector accounting for 32.6% of the real-time decision-making AI agents market share in 2024, according to market research. With a current valuation of $3.6 billion, this market is projected to reach $144.9 billion by 2034, representing a compound annual growth rate of 44.7%.
In healthcare, AI is revolutionizing diagnostic capabilities and treatment planning. A recent study published in Inc.com found that K Health’s diagnostic AI model matched doctors’ diagnoses in about 68% of cases, and in the remaining third, expert reviewers rated the AI’s treatment plan as superior. This demonstrates AI’s potential to enhance medical decision-making, though it doesn’t eliminate the need for human doctors.
Success Rates and Effectiveness
The effectiveness of AI in decision-making varies significantly across industries and applications. A study by Infosys found that only 19% of AI use cases fully deliver on their business objectives, while another 32% show partial success, as reported by Technology Magazine. This suggests that while AI has tremendous potential, implementation challenges remain.
In healthcare, AI models can predict heart attacks with up to 90% accuracy, enabling timely interventions, according to Entefy. Meanwhile, in financial services, 91% of firms have implemented AI or have plans to do so, highlighting its strategic importance.
A particularly promising area is predictive AI in manufacturing and retail, where it enhances demand forecasting and inventory management. For example, predictive AI-driven anomaly detection for a global industrial tech client delivered a 650% ROI, according to Grid Dynamics.
The Human-AI Partnership
Despite these impressive statistics, the most effective implementations of AI decision-making systems involve human-AI collaboration rather than complete automation.
“Trust in AI has to start before the first line of code is written,” emphasizes Reggie Townsend, Vice President of the SAS Data Ethics Practice. This highlights the need for ethical frameworks to ensure AI systems are fair and transparent, especially in critical industries where AI can amplify biases if not properly governed.
In manufacturing, Roque Martin, CEO of Aras, emphasizes the importance of AI in product development, stating, “Incorporating AI into product development isn’t just about keeping up – it’s about staying ahead.” This perspective from Plant Services highlights how AI helps manufacturers design smarter products while managing intellectual property and ensuring regulatory compliance.
The Regulatory Landscape
As AI decision-making becomes more prevalent, regulatory frameworks are evolving to ensure responsible deployment. The European Union’s Artificial Intelligence Act (AIA) is a comprehensive framework that includes rules for high-risk AI systems, such as those used in healthcare and transportation. It requires transparency, human oversight, and impact assessments for AI systems that significantly impact individuals, as reported in Frontiers in Political Science.
China has proposed the Artificial Intelligence Law, which would impose legal requirements on AI developers and deployers, particularly for high-risk systems. The Cyberspace Administration of China (CAC) has implemented stricter rules on AI-generated content, including mandatory watermarking systems, according to Mind Foundry.
In the United States, the approach to AI regulation is more fragmented, with various state-specific policies. For example, Texas and California have introduced legislation to govern AI use in decision-making processes. The Securities and Exchange Commission (SEC) published an AI Compliance Plan in September 2024 to manage AI risks in financial markets, as noted by Sidley.
Ethical Considerations and Governance
Ensuring responsible AI decision-making requires robust ethical frameworks and governance models. Key principles include fairness and non-discrimination, transparency and explainability, accountability and governance, privacy protection, and security.
Google’s PAIR (People and AI Research) initiative focuses on building human-centered AI systems. It includes tools like the What-If Tool for model performance and Model Cards for transparency. These tools help stakeholders understand AI models’ intended use, limitations, and ethical considerations, enhancing accountability and trust, as reported by LogicGate.
In healthcare, ethical AI frameworks ensure systems are fair, transparent, and compliant with privacy regulations, reducing diagnostic biases and enhancing patient outcomes. Similarly, in finance, responsible AI ensures processes like fraud detection and loan approvals are unbiased, promoting financial inclusion and transparency in decision-making, according to Convin.
Accessibility and Equity Challenges
AI decision-making systems present significant challenges in ensuring accessibility and equity across different demographics. The digital divide is a major concern, as AI technologies often require access to digital resources, which can exacerbate existing inequalities. Many populations, particularly in the Global South and marginalized communities, lack the financial means, education, or digital literacy necessary to benefit from AI-driven innovations.
For instance, women’s access to mobile internet in India still lags behind men’s, limiting their ability to use AI-based services, as reported by the Hindustan Times. Similarly, AI models can inherit biases from their training data, leading to unfair outcomes. For example, 44% of AI models exhibit gender bias, which can have devastating real-world consequences, such as making it harder for women to access loans.
The Economic Impact and Job Market
The economic implications of AI decision-making are profound. According to the United Nations Conference on Trade and Development (UNCTAD), AI is on track to reach a staggering $4.8 trillion in market value by 2033, comparable to the size of Germany’s economy. However, its benefits remain unequally distributed, as reported by Tekedia.
UNCTAD estimates that AI could impact up to 40% of jobs globally, raising concerns about widespread job displacement. This displacement is not limited to traditional blue-collar roles but is increasingly shifting toward white-collar professions as AI systems grow more sophisticated. To address these challenges, the UN is calling for proactive labor policies, including investing in reskilling, upskilling, and workforce adaptation.
Essential Skills for the AI Era
As AI decision-making systems become increasingly integral to critical industries, professionals must develop specific skills and competencies to work effectively alongside these technologies.
Data literacy is crucial for interpreting and making decisions based on AI outputs. This includes understanding data analysis, machine learning concepts, and AI ethics. Companies like Goldman Sachs and JPMorgan Chase are using AI to enhance trading strategies and risk management, highlighting the need for data-driven decision-making skills, according to Entefy.
The ability to make decisions in tandem with AI recommendations is also essential. Gartner predicts that by 2028, 15% of day-to-day work decisions will be made autonomously by Agentic AI systems, highlighting the need for professionals to work effectively with these systems, as reported by Elnion.
Additionally, as AI automates routine tasks, professionals must focus on complex problem-solving and adaptability. This includes being creative and proactive in addressing issues that AI systems cannot handle, as noted by IT Wire.
Looking Ahead: The Future of AI Decision-Making
The future of AI decision-making in critical industries will likely involve increased autonomy balanced with human oversight. According to a recent survey by PagerDuty, 51% of companies are already leveraging AI agents, and 94% believe they will adopt agentic AI more quickly than generative AI. Furthermore, 62% of respondents anticipate triple-digit ROI from agentic AI implementations, as reported by Street Insider.
The survey also found that leaders expect nearly 40% of work to be automated or expedited with the help of AI agents. However, companies are learning from past experiences with generative AI and are prioritizing training for agentic AI, with 61% planning organization-wide seminars or structured initiatives.
Practical Applications: Success Stories
One compelling example of AI decision-making in action comes from the National Institute on Drug Abuse (NIDA), which recently reported on an AI-driven screening tool for opioid use disorder. The tool successfully identified hospitalized adults at risk and recommended referrals to inpatient addiction specialists. Compared to patients who received provider-initiated consultations, patients with AI screening had 47% lower odds of being readmitted to the hospital within 30 days after their initial discharge. This reduction in readmissions translated to nearly $109,000 in estimated healthcare savings during the study period, as reported by NIDA.
Another success story comes from the cybersecurity sector, where Quantinuum’s Quantum Origin has become the first software Quantum Random Number Generator (QRNG) to receive National Institute of Standards and Technology (NIST) validation. This achievement establishes it as a crucial tool for federal agencies and agency partners in their mandated migration to post-quantum cryptography under National Security Memorandum 10, as reported by the Portland Tribune.
Balancing Act: Human Judgment and AI Efficiency
The most effective approach to AI decision-making in critical industries appears to be a balanced partnership between human judgment and AI efficiency. While AI can process vast amounts of data and identify patterns beyond human capability, human oversight ensures ethical considerations, contextual understanding, and accountability.
This balanced approach is particularly important in healthcare, where AI can enhance diagnostic accuracy but should not replace the human touch in patient care. In finance, AI can improve risk assessment and fraud detection, but human judgment is essential for understanding complex market dynamics and customer needs.
What This Means For You
For professionals across industries, the rise of AI decision-making systems presents both challenges and opportunities. To stay relevant and effective in this evolving landscape, consider the following actions:
- Invest in AI literacy: Understand the basics of how AI systems work, their capabilities, and limitations. This knowledge will help you collaborate effectively with AI tools and make informed decisions about their implementation.
- Develop complementary skills: Focus on developing skills that AI cannot easily replicate, such as creativity, empathy, ethical judgment, and complex problem-solving. These human capabilities will remain valuable even as AI becomes more sophisticated.
- Advocate for responsible AI: Regardless of your role, advocate for ethical AI deployment in your organization. This includes ensuring diverse representation in AI development teams, regular auditing for bias, and maintaining human oversight of critical decisions.
- Prepare for continuous learning: The AI landscape is evolving rapidly. Commit to continuous learning and skill development to stay ahead of the curve and adapt to changing job requirements.
Pro Tip:
When evaluating AI decision-making systems for your organization, look beyond the technical specifications. Consider how the system will integrate with existing workflows, how it will be governed, and what training your team will need to use it effectively.
Conclusion
AI decision-making in critical industries represents one of the most significant technological shifts of our time. When implemented thoughtfully, with appropriate human oversight and ethical considerations, these systems can enhance efficiency, accuracy, and outcomes across sectors. However, the human element remains irreplaceable, providing the judgment, ethics, and contextual understanding that AI currently lacks.
As we navigate this new frontier, the most successful organizations will be those that strike the right balance between leveraging AI’s capabilities and preserving human judgment in critical decisions. This balanced approach will not only maximize the benefits of AI but also mitigate its risks, ensuring that technology serves humanity rather than the other way around.
What has been your experience with AI decision-making systems in your industry? Share your thoughts and join the conversation about how we can harness AI’s potential while maintaining the essential human element in critical decisions.